IS THE INDIAN YOUNG POPULATION'S USAGE OF GENERATIVE AI THE ACADEMIC DRIVER FOR THE NEXT DECADES?
DOI:
https://doi.org/10.14456/aelr.2026.1Keywords:
Artificial Intelligence, Generative AI, Youth, India, Ethical ConsiderationsAbstract
This qualitative study investigates the extent and nature of generative AI usage among young Indians for academic purposes. Employing a thematic analysis of data collected from 206 participants aged 16-30, the research explores the motivations driving this adoption, examining the shift from traditional research methods towards AI-powered tools. Findings reveal a high level of awareness and frequent usage of generative AI across various platforms, primarily driven by convenience, efficiency, and ease of access. While participants express limited concern regarding ethical implications, the study highlights the potential for over-reliance and the need for greater awareness of responsible AI usage. The research contributes to a growing body of work examining the impact of generative AI on young people, emphasizing the need for responsible adoption strategies, particularly in educational settings. Further quantitative research with larger, more representative samples is recommended to corroborate and extend these initial qualitative findings.
References
Brown, T., Mann, B., Ryder, N., Subbiah, M., Kaplan, Dhariwal, P., Neelakantan, A., Shyam, P., Sastry, G., Askell, A., Agarwal, S., Herbert-Voss, A., Krueger, G., Henighan, T., Child, R., Ramesh, A., Ziegler, D., Wu, J., Winter, C., Hesse, C., Chen, M., Sigler, E., Litwin, M., Gray, S., Chess, B., Clark, J., Berner, C., McCandlish, S., Radford, A., Sutskever, I., & Amodei, D. (2020). Language Models are Few-Shot Learners. Retrieved from https://arxiv.org/abs/2005.14165.
Christie's. (2018). Unmistakable and the interface between art and artificial intelligence. Retrieved from www.christies.com/en/stories/a-collaboration-between-two-artists-one-human-one-a-machine-0cd01f4e232f4279a525a446d60d4cd1.
Donahue, J., Krähenbühl, P., & Darrell, T. (2017). Adversarial Feature Learning. arXiv https://arxiv.org/abs/1605.09782.
Fadnavis, N., Patil, G., Padyana, U., Rai, H., & Ogeti, P. (2023). The Role of Generative Adversarial Networks in Transforming Creative Industries: Innovations and Implications. International Journal of Intelligent Systems and Applications in Engineering, 11(6s), 849-855.
Gallegos, I., Rossi, R., Barrow, J., Tanjim, M., Kim, S., Dernoncourt, F., … Ahmed, N. (2024). Bias and Fairness in Large Language Models: A Survey. arXiv https://arxiv.org/abs/2309.00770v3.
Gatys, L., Ecker, A., & Bethge, M. (2016). Image Style Transfer Using Convolutional Neural Networks. A paper presented at the 2016 IEEE Conference on Computer Vision and Pattern Recognition, Las Vegas, USA.
Goodfellow, I., Pouget-Abadie, J., Mirza, M., Xu, B., Warde-Farley, D., Ozair, S., Courville, A., & Bengio, Y. (2014). Generative Adversarial Nets. A paper presented at the Advances in Neural Information Processing Systems 27, Montreal, Canada.
Habib, S., Vogel, T., Anli, X., & Thorne, E. (2024). How does generative artificial intelligence impact student creativity?. Journal of Creativity, 34(1), 100072.
Hadjeres, G., Pachet, F., & Nielsen, F. (2017). DeepBach: A Steerable Model for Bach Chorales Generation. Retrieved from https://arxiv.org/abs/1612.01010.
Hanna, M., Pantanowitz, L., Jackson, B., Palmer, O., Visweswaran, S., Pantanowitz, J., … Rashidi, H. (2025). Ethical and Bias Considerations in Artificial Intelligence/Machine Learning. Modern Pathology, 38(3), 100686.
Hao, K. (2019). The biggest threat of deepfakes isn’t the deepfakes themselves. Retrieved from www.technologyreview.com/2019/10/10/132667/the-biggest-threat-of-deepfakes-isnt-the-deepfakes-themselves/.
Hassija, V., Chamola, V., Mahapatra, A., Singal, A., Goel, D., Huang, K., … Hussain, A. (2024). Interpreting Black-Box Models: A Review on Explainable Artificial Intelligence. Cognitive Computation, 16, 45-74.
Huang, C., Zhang, Z., & Mao, B., & Yao, X. (2023). An Overview of Artificial Intelligence Ethics. IEEE Transactions on Artificial Intelligence, 4(4), 799-819.
Karras, T., Laine, S., & Aila, T. (2019). A Style-Based Generator Architecture for Generative Adversarial Networks. A paper presented at the 2019 IEEE/CVF Conference on Computer Vision and Pattern Recognition, Long Beach, USA.
Kingma, D., & Welling, M. (2013). Auto-Encoding Variational Bayes. Retrieved from https://arxiv.org/abs/1312.6114.
Larsen, A., Sønderby, S., Larochelle, H., & Winther, O. (2016). Autoencoding Beyond Pixels Using a Learned Similarity Metric. Retrieved from https://arxiv.org/abs/1512.09300.
Lv, Z. (2023). Generative Artificial Intelligence in the Metaverse Era. Cognitive Robotics, 3, 208-217.
Mittelstadt, B., Allo, P., Taddeo, M., Wachter, S., & Floridi, L. (2016). The ethics of algorithms: Mapping the debate. Big Data & Society, 3(2). https://doi.org/10.1177/2053951716679679.
Ribeiro, M., Singh, S., & Guestrin, C. (2016). "Why Should I Trust You?": Explaining the Predictions of Any Classifier. A paper presented at the 22nd ACM SIGKDD International Conference on Knowledge Discovery and Data Mining, San Francisco, USA.
Schmidhuber, J. (2015). Deep Learning in Neural Networks: An Overview. Neural Networks, 61, 85-117.
Segler, M., Kogej, T., Tyrchan, C., & Waller, M. (2018). Generating Focused Molecule Libraries for Drug Discovery with Recurrent Neural Networks. ACS Central Science, 4(1), 120-131.
Tripathi, S., Augustin, A,. Dunlop, A., Sukumaran, R., Dheer, S., Zavalny, A., … Kim, E. (2022). Recent advances and application of generative adversarial networks in drug discovery, development, and targeting. Artificial Intelligence in the Life Sciences, 2, 100045.
Wolterink, J., Leiner, T., Viergever, M., & Išgum, I. (2017). Generative Adversarial Networks for Noise Reduction in Low-Dose CT. IEEE Transactions on Medical Imaging, 36(12), 2536-2545.
Yang, L., Zhang, Z., Song, Y., Hong, S., Xu, R., Zhao, Y., … Yang, M. (2024). Diffusion Models: A Comprehensive Survey of Methods and Applications. arXiv https://arxiv.org/abs/2209.00796.
Yang, Z., Hu, Z., Dyer, C., Xing, E., & Berg-Kirkpatrick, T. (2018). Unsupervised Text Style Transfer Using Language Models as Discriminators. Paper presented at the 32nd Conference on Neural Information Processing Systems (NeurIPS 2018), Montréal, Canada.
Zhang, T. (2022). Deepfake generation and detection, a survey. Multimedia Tools and Applications, 81, 6259-6276.
Zhao, J., Li, M., & Wang, W. (2021). The Ethical Implications of Using Biased Data in Training Generative AI Models. Ethics and Information Technology, 23(3), 259-273.
Zhu, J., Park, T., Isola, P., & Efros, A. (2017). Unpaired Image-to-Image Translation Using Cycle-Consistent Adversarial Networks. A paper presented at the 2017 IEEE International Conference on Computer Vision, Venice, Italy.
Zhu, K., Liu, X., & Yang, H. (2018). A Survey of Generative Adversarial Networks. Paper presented at the 2018 Chinese Automation Congress (CAC), Xi'an, China.
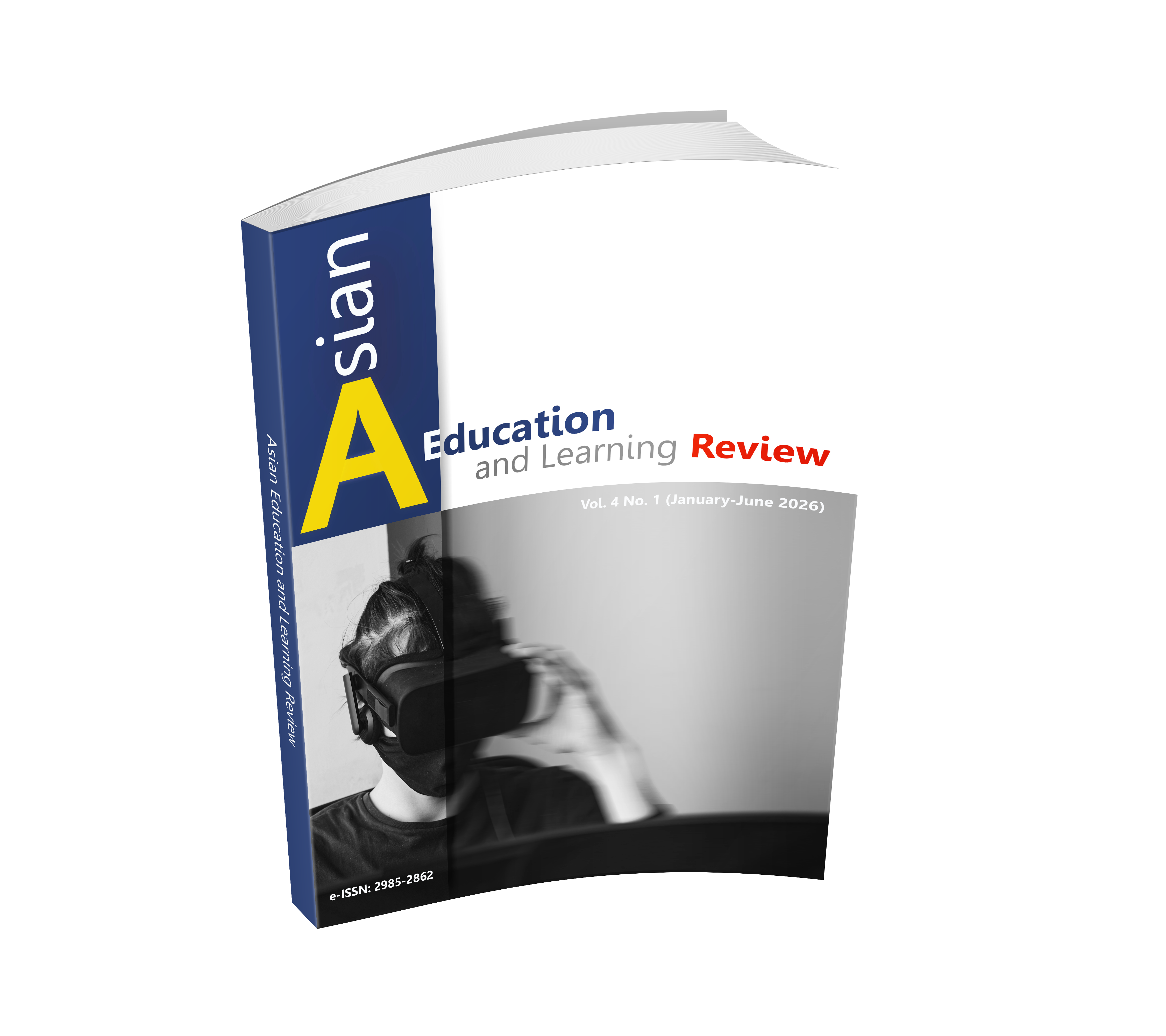
Downloads
Published
How to Cite
Issue
Section
License
Copyright (c) 2025 Authors

This work is licensed under a Creative Commons Attribution-NonCommercial-NoDerivatives 4.0 International License.