ARTIFICIAL INTELLIGENCE: A CATALYST FOR SUSTAINABLE EFFECTIVENESS IN COMPULSORY EDUCATION MANAGEMENT
DOI:
https://doi.org/10.14456/aelr.2025.4Keywords:
Artificial Intelligence, Compulsory Education Management, Adaptive Learning Systems, Automated Administrative Tasks, Intelligent Tutoring SystemsAbstract
This article investigates the application of artificial intelligence (AI) in compulsory education, highlighting its potential to revolutionize learning and improve student outcomes. AI-driven adaptive systems offer personalized learning experiences tailored to individual student needs, promising to reduce achievement gaps and ensure equitable access to quality education. Furthermore, AI-powered analytics provide valuable insights for educators, enabling early identification of learning difficulties and the implementation of targeted interventions. This data-driven approach can increase graduation rates, enhance academic performance, and optimize resource allocation. Beyond immediate improvements, AI's integration prepares students for a future that is increasingly reliant on technology. By focusing on uniquely human skills—critical thinking and problem-solving—while AI handles routine tasks, educational systems can better equip students for success in a complex, technology-driven world. However, successful AI integration requires addressing critical challenges. These include developing robust infrastructure, providing ongoing professional development for educators, and establishing transparent governance frameworks to address concerns regarding data privacy and algorithmic bias. Ultimately, a collaborative and proactive approach from policymakers and educators is essential to harness AI's transformative potential responsibly and equitably.
References
Baker, B., Di Carlo, M., & Weber, M. (2020). The adequacy and fairness of state school finance systems. Washington, D.C.: Albert Shanker Institute.
Baker, R., & Hawn, A. (2021). Algorithmic bias in education. International Journal of Artificial Intelligence in Education, 31(4), 1-14.
Baker, R., & Inventado, P. (2014). Educational data mining and learning analytics. In Learning analytics (pp. 61-75). New York: Springer.
Baker, T., Smith, L., & Anissa, N. (2019). Educ-AI-tion rebooted? Exploring the future of artificial intelligence in schools and colleges. London: Nesta Foundation.
Bakhshaei, M., Jochim, A., & Stelitano, L. (2021). Personalized Learning in Practice: A District-Level View. California: RAND Corporation.
Bates, A. (2015). Teaching in a Digital Age: Guidelines for Designing Teaching and Learning. Vancouver: Tony Bates Associates Ltd.
Bienkowski, M., Feng, M., & Means, B. (2012). Enhancing teaching and learning through educational data mining and learning analytics: An issue brief. Washington, D.C.: US Department of Education, Office of Educational Technology.
Bingham, A., & Solverson, N. (2016). Using data mining to explore why community college transfer students earn bachelor's degrees. Research in Higher Education, 57(2), 170-195.
Borenstein, J., & Howard, A. (2021). Emerging challenges in AI and the need for AI ethics education. AI and Ethics, 1(1), 61-65.
Bughin, J., Hazan, E., Lund, S., Dahlström, P., Wiesinger, A., & Subramaniam, A. (2018). Skill shift: Automation and the future of the workforce. New York: McKinsey Global Institute.
Care, E., Griffin, P., Wilson, M., & Csapó, B. (2018). Assessment and teaching of 21st century skills. Dordrecht: Springer.
Chatterjee, P., & Bhattacharya, S. (2020). Adoption of artificial intelligence in higher education: A quantitative analysis using structural equation modelling. Education and Information Technologies, 25(5), 3443-3463.
Chen, L., Chen, P., & Lin, Z. (2020). Artificial intelligence in education: A review. IEEE Access, 8, 75264-75278.
Conati, C., Gertner, A., & VanLehn, K. (2002). Using Bayesian networks to manage uncertainty in student modeling. User Modeling and User-Adapted Interaction, 12(4), 371-417.
Darling-Hammond, L., Flook, L., Cook-Harvey, C., Barron, B., & Osher, D. (2020). Implications for educational practice of the science of learning and development. Applied Developmental Science, 24(2), 97-140.
Darling-Hammond, L., Hyler, M., & Gardner, M. (2017). Effective Teacher Professional Development. California: Learning Policy Institute.
de Brito, S., Silva, A., Cruz, A., Monteiro, M., Vijaykumar, N., Silva, M., Costa, J., & Francês, C. (2020). Concentration of access to information and communication technologies in the municipalities of the Brazilian legal Amazon. PloS one, 15(4), e0232395.
Farrell, G., & Sidorko, P. (2019). Automate this: An investigation into the use of robotic process automation in academic libraries. Library Management, 40(8/9), 521-530.
Haßler, B., Major, L., & Hennessy, S. (2018). Tablet use in schools: A critical review of the evidence for learning outcomes. Journal of Computer Assisted Learning, 34(2), 174-187.
Holmes, W., Bialik, M., & Fadel, C. (2019). Artificial Intelligence in Education: Promises and Implications for Teaching and Learning. Massachusetts: Center for Curriculum Redesign.
Hwang, G., Yeh, T., Hung, C., & Wang, S. (2020). An integrated model for developing adaptive learning systems based on learning/cognitive styles and its application to English as a foreign language learning. Computers & Education, 154, 103907.
Ingersoll, R., & Collins, G. (2017). Participation in the learning-centered leadership program. Andhra Pradesh: GSE Publications.
Jayaprakash, S., Moody, E., Lauría, E., Regan, J., & Baron, J. (2014). Early alert of academically at-risk students: An open source analytics initiative. Journal of Learning Analytics, 1(1), 6-47.
Klein, C., Dalton, D., & Takemura, T. (2020). Balancing privacy and utility in cross-university learning analytics: A case study of the UniversityNow learning analytics database. Computers & Education, 151, 103879.
Kulik, J., & Fletcher, J. (2016). Effectiveness of intelligent tutoring systems: A meta-analytic review. Review of Educational Research, 86(1), 42-78.
Luckin, R. (2017). Towards artificial intelligence-based assessment systems. Nature Human Behaviour, 1(0028), 1-3.
Luckin, R., Holmes, W., Griffiths, M., & Forcier, L. (2016). Intelligence Unleashed: An argument for AI in Education. London: Pearson.
Lupton, D., & Williamson, B. (2017). The datafied child: The dataveillance of children and implications for their rights. New Media & Society, 19(5), 780-794.
Makransky, G., & Petersen, G. (2021). The cognitive affective model of immersive learning (CAMIL): A theoretical research-based model of learning in immersive virtual reality. Educational Psychology Review, 33(3), 937-958.
Mandinach, E., & Gummer, E. (2016). Data literacy for educators: Making it count in teacher preparation and practice. New York: Teachers College Press.
Nye, B. (2015). Intelligent tutoring systems by and for the developing world: a review of trends and approaches for educational technology in a global context. International Journal of Artificial Intelligence in Education, 25(2), 177-203.
Ocepek, U., Rugelj, J., & Bosnić, Z. (2021). Exploring the potential of chatbots in higher education. International Journal of Emerging Technologies in Learning, 16(14), 4-20.
OECD. (2017). The Funding of School Education: Connecting Resources and Learning. Paris: OECD Publishing.
OECD. (2018). Education at a Glance 2018: OECD Indicators. Paris: OECD Publishing.
OECD. (2019a). OECD Future of Education and Skills 2030: OECD Learning Compass 2030. Paris: OECD Publishing.
OECD. (2019b). Personalised Learning: A New ICT-Enabled Education Approach. Paris: OECD Publishing.
OECD. (2020). TALIS 2018 Results (Volume II): Teachers and School Leaders as Valued Professionals. Paris: OECD Publishing.
OECD. (2021). Digital Education Outlook 2021: Pushing the Frontiers with Artificial Intelligence, Blockchain and Robots. Paris: OECD Publishing.
Pane, J., Griffin, B., McCaffrey, D., & Karam, R. (2014). Effectiveness of Cognitive Tutor Algebra I at scale. Educational Evaluation and Policy Analysis, 36(2), 127-144.
Pane, J., Steiner, E., Baird, M., & Hamilton, L. (2017). Promising Evidence on Personalized Learning. California: RAND Corporation.
Pangrazio, L., & Sefton-Green, J. (2020). The social utility of 'data literacy'. Learning, Media and Technology, 45(4), 412-426.
Papamitsiou, Z., & Economides, A. (2014). Learning analytics and educational data mining in practice: A systematic literature review of empirical evidence. Educational Technology & Society, 17(4), 49-64.
Popenici, S., & Kerr, S. (2017). Exploring the impact of artificial intelligence on teaching and learning in higher education. Research and Practice in Technology Enhanced Learning, 12(1), 1-13.
Porayska-Pomsta, K., & Rajendran, G. (2019). Accountability in algorithmic decision-making: A view from computational creativity in education. In Creativity and Universality in Language (pp. 123-139). Cham: Springer.
Reich, J., & Ito, M. (2017). From good intentions to real outcomes: Equity by design in learning technologies. California: Digital Media and Learning Research Hub.
Roll, I., & Wylie, R. (2016). Evolution and revolution in artificial intelligence in education. International Journal of Artificial Intelligence in Education, 26(2), 582-599.
Santoro, D. (2018). Demoralized: Why teachers leave the profession they love and how they can stay. Massachusetts: Harvard Education Press.
Scherer, R. (2016). Learning from the past: Directions for the design of adaptive educational systems. International Journal of Artificial Intelligence in Education, 26(2), 709-749.
Selwyn, N. (2017). Education and Technology: Key Issues and Debates. 2nd ed. London: Bloomsbury Academic.
Shermis, M., & Burstein, J. (eds.). (2013). Handbook of automated essay evaluation: Current applications and new directions. London: Routledge.
Shute, V., & Rahimi, S. (2017). Review of computer‐based assessment for learning in elementary and secondary education. Journal of Computer Assisted Learning, 33(1), 1-19.
Sottilare, R., Graesser, A., Hu, X., & Holden, H. (2013). Design recommendations for intelligent tutoring systems (Vol. 1). Maryland: US Army Research Laboratory.
Southgate, E., Blackmore, K., Pieschl, S., Grimes, S., McGuire, J., & Smithers, K. (2019). Artificial intelligence and emerging technologies in schools. Canberra: Australian Government Department of Education.
Tan, C., Chua, Y., & Goh, O. (2019). Artificial intelligence in Singapore schools: Learning about and with artificial intelligence. Asian Journal of the Scholarship of Teaching and Learning, 9(2), 173-192.
UNESCO. (2015). Education 2030: Incheon Declaration and Framework for Action for the implementation of Sustainable Development Goal 4. Paris: UNESCO.
UNESCO. (2020a). Education in a post-COVID world: Nine ideas for public action. Paris: UNESCO.
UNESCO. (2020b). Global Education Monitoring Report 2020: Inclusion and education: All means all. Paris: UNESCO.
UNESCO. (2021). AI and education: Guidance for policymakers. Paris: UNESCO.
VanLehn, K. (2011). The relative effectiveness of human tutoring, intelligent tutoring systems, and other tutoring systems. Educational Psychologist, 46(4), 197-221.
Voulgaris, G., & Lekkas, Z. (2021). Opportunities and Challenges of Intelligent Automation in Higher Education Administration. European Journal of Engineering and Technology Research, 6(2), 18-24.
Williamson, B., & Piattoeva, N. (2019). Objectivity as standardization in data-scientific education policy, technology and governance. Learning, Media and Technology, 44(1), 64-76.
Williamson, D., Mislevy, R., & Bejar, I. (eds.). (2006). Automated scoring of complex tasks in computer-based testing. New Jersey: Lawrence Erlbaum Associates Publishers.
Zawacki-Richter, O., Marín, V., Bond, M., & Gouverneur, F. (2019). Systematic review of research on artificial intelligence applications in higher education - where are the educators?. International Journal of Educational Technology in Higher Education, 16(1), 39.
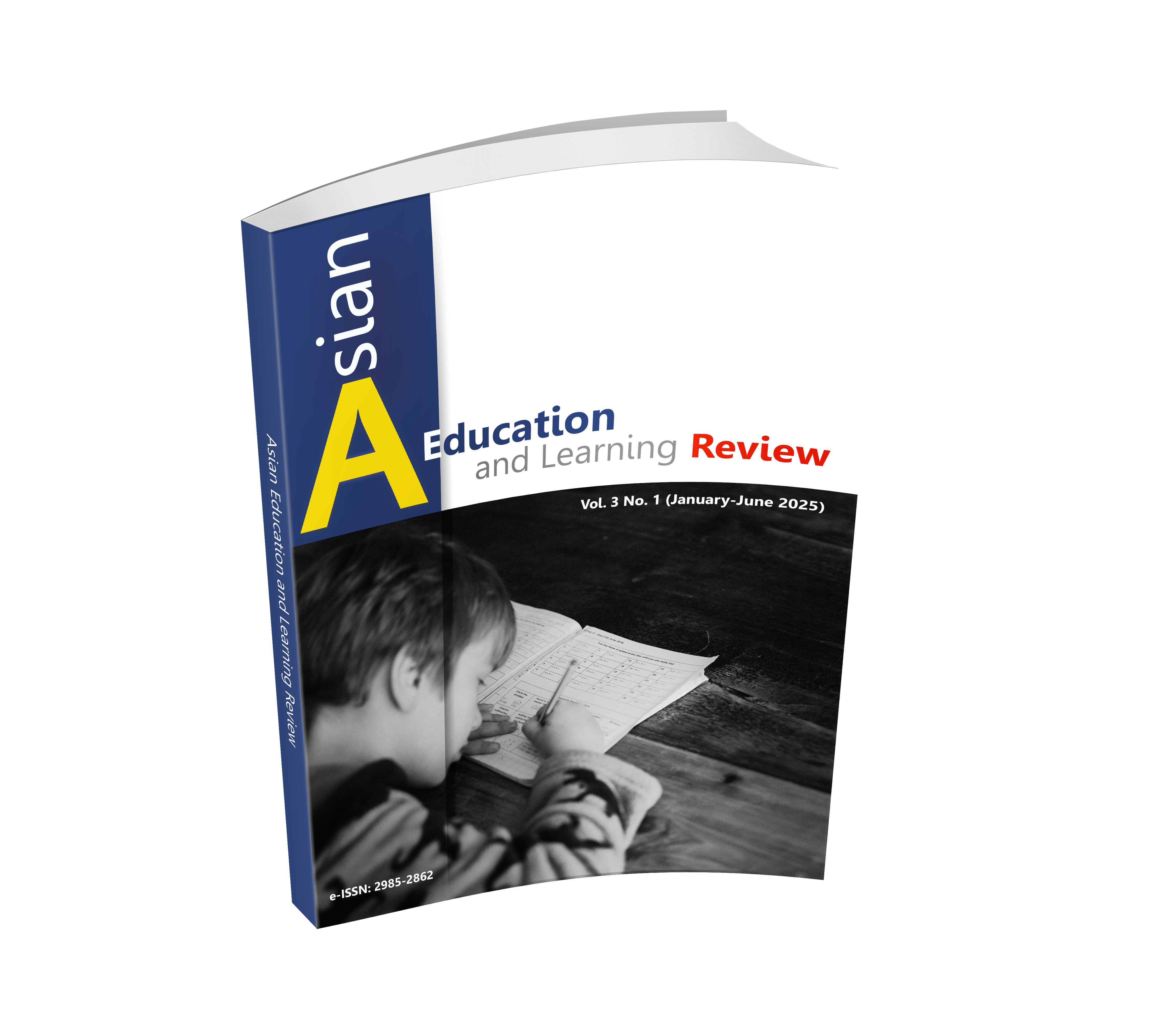
Downloads
Published
How to Cite
Issue
Section
License
Copyright (c) 2025 Authors

This work is licensed under a Creative Commons Attribution-NonCommercial-NoDerivatives 4.0 International License.